3 Keys to Enhance Your Online Customer Journey with AI
How artificial intelligence can deliver a personalized online experience.
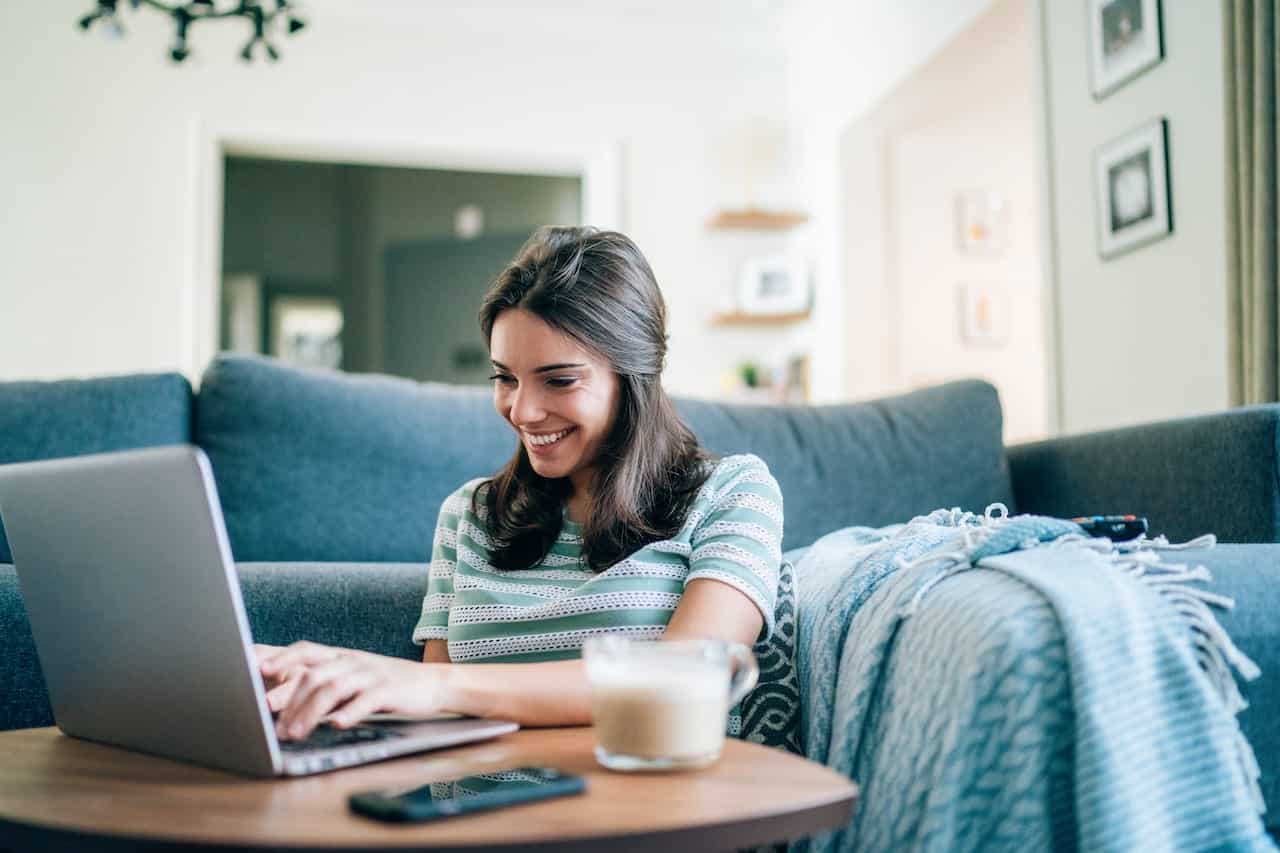
This post was originally published at Retail Touchpoints.
Gartner reported that COVID-19 has accelerated permanent changes in retail customer behavior. Companies that can’t deliver a seamless, personalized online experience risk losing customers and revenue. Now more than ever, retailers are in a race to reinvent their digital playbooks to stay competitive. Fortunately, with practical artificial intelligence (AI), retailers can dramatically improve the online customer journey at scale through frictionless product discovery and virtual interactions. In this blog, I’ll dive into three ways AI can help you deliver.
1. Signals are king.
Signals are all interactions and data points unique to a customer, including search queries, clicks, add-to-cart, checkout, profile information, purchase history, etc. You can capture web-based signals as well as offline activities and interactions outside of your ecommerce platform. Many retailers have successfully used signals to enhance the customer experience, and if you’re not getting value from signals your business is at a competitive disadvantage.
Now, signals that are important to a certain business may not be to another. For example, to an auto parts retailer, it’s important to know if a certain part is being returned frequently — a sign that the part’s description may be lacking or inaccurate. On the other hand, for personalized engravement or intimate apparel retailers, products are often non-returnable and so returns aren’t very useful as a signal. Because signals are unique to each customer and each business, they will be key to helping your AI algorithms unlock an individual customer’s behavior and reduce friction along his or her online journey.
AI can perform even better with historical signal data. Our customers often ask “How long does it take for your AI system to learn about customer behavior?” Two weeks? Maybe six? How about right away? It’s possible. You can use historically available clickstream data — the raw signals about customers — to train your AI-powered ecommerce platform to understand customer intent from day one.
2. Machine learning powers search relevance at scale.
Do you have teams of search analysts or curators doing manual reviews of poorly-performing searches and manually boosting their relevance? If so, you understand how painful, resource-gouging and unscalable this is, especially when your product assortment and consumer behavior change. This also means that you need to have a lot of failed experience data to make search better.
Manually tuning thousands of zero-result and poor-performing searches takes a long time in the first place, and once you’ve put in a manual curation rule, you have to maintain it as time goes on. It takes away valuable time that your team could’ve spent on other business priorities. This is where machine learning shines — it provides a nice balance between deterministic rules and complete automation.
ML-based vector search gives us a shot at good site search the first time someone searches outside the boundaries of our vocabulary assortment. Vector search places concepts with similar purposes in the same semantic space, helping shoppers discover products often used together in a natural and organic way. Vector search is especially powerful in delivering intuitive search relevance and eliminating the much dreaded zero results. For example, vector search helped our customer — a top-five global retailer — reduce zero-result searches by 91% and increase average order value by 28%.
3. Chatbots are your support agents’ friends.
What’s cool about vector search is that it can also enhance customer service chatbots, improving customer satisfaction while easing the burden on your customer service agents. Given the pandemic-driven upward trend in online shopping, good onsite customer support is more critical than ever. According to Forrester’s “Must-Have E-commerce Features” report, “In 2019, 27% of U.S. online adults said it was important for a retailer to offer a live chat service on the website; in 2020, 42% of U.S. online adults said it was important for a retailer to offer live online chat to ask questions and get support on the website.” That’s a whopping 15-percentage-point increase in customer demand for online chat service in just one year.
Chatbots built on conversational AI have the ability to not only provide answers but also ask questions to guide customers to the right products. The forefront of retailer chatbot innovation is a “product finder” — a structured product discovery workflow in which the app asks you questions and guides you to the product recommendations that it thinks work best for you. I believe finders can propel chatbots to the next level to deliver the same in-store service experience that customers enjoy online.
LEARN MORE
Contact us today to learn how Lucidworks can help your team create powerful search and discovery applications for your customers and employees.