User Intent Steers AI-Powered Search
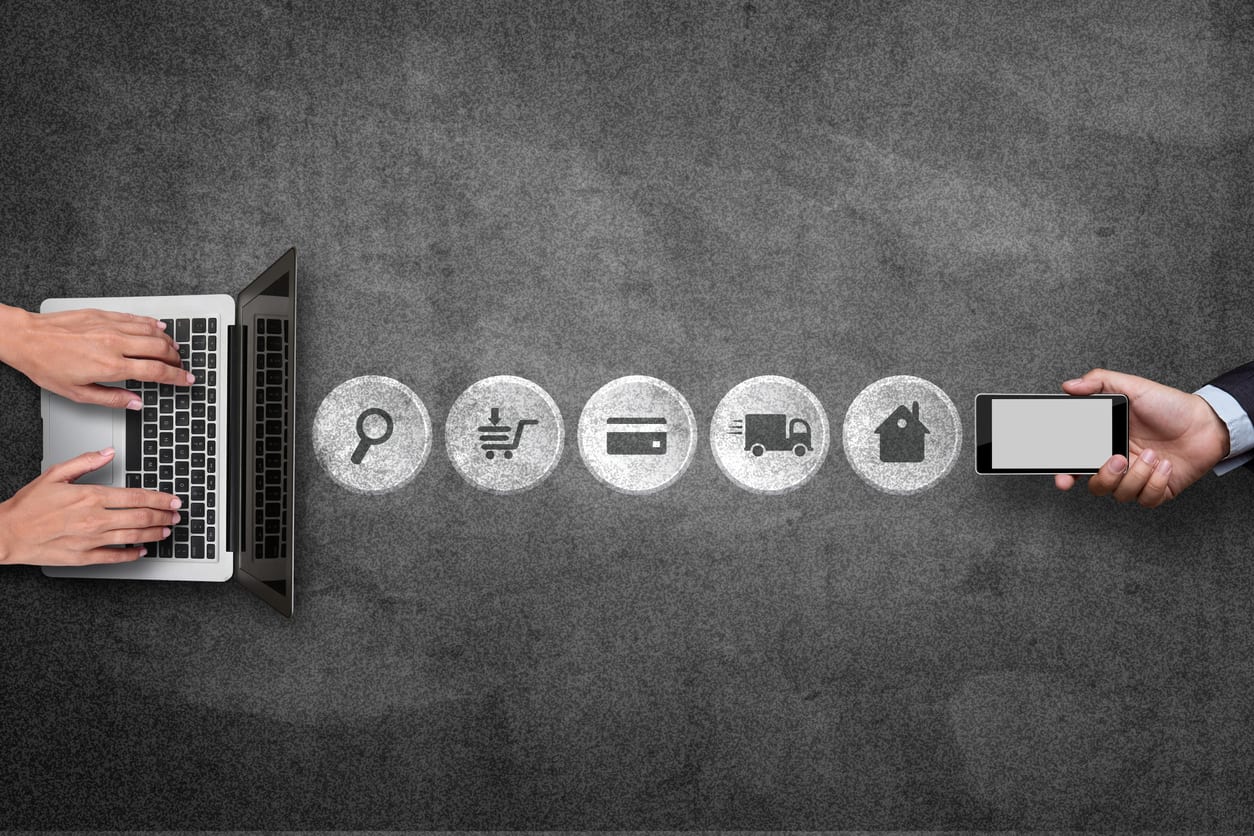
Consumers expect personalized experiences on retail sites. After all, they get them on social media, in entertainment, on mobile devices, and while they search the web — why settle for less when shopping?
Marketers agree. A recent survey of 700 U.S. marketers conducted by ClickZ and Chatmeter found that fully half of respondents identified “changing customer behavior driven by new technology” as the number one search trend of 2019.
In ecommerce, the shopper’s search experiences can vary widely. Amazon’s search and recommendation engines, powered by artificial intelligence (AI) and machine learning (ML), provide highly relevant and personalized results based on a shopper’s prior purchases and browsing, among other factors, but the search experience on most other retail sites is often disappointing.
The situation is beginning to change, though. Trends in distributed computing and open-source programming are making it possible for organizations without enormous scale and resources to dramatically improve ecommerce search and recommendations for shoppers — when they work with the right partner.
To find out why search is challenging from a technical perspective and how that’s changing, Lucidworks tapped the expertise of Peter Curran, president and cofounder of Cirrus10 and a consultant on the business and technology of ecommerce, in a question-and-answer session.
Curran has been a featured speaker in two recent Lucidworks webinars, Why Your Customers Can’t Find What They Are Looking For and Create the Ideal Customer Experience—Without Giving Up Control. He has overseen the implementation of Lucidworks’ Fusion search application with three clients, but he also works with other solution providers and on projects other than search.
Q: What technologies are making it possible for ecommerce companies to improve their search significantly?
A: With the emergence of ML and distributed computing, companies can do things they couldn’t do before without writing a bunch of rules. For example, Lucidworks Fusion has a distributed computing architecture based on Apache Spark that is integrated with a search platform. Distributed computing allows search engines to handle more scenarios algorithmically, and Fusion can do a lot of complicated ML calculations that would be too computationally expensive without this marriage.
Q: Why has search been such a challenge for e-retailers?
A: E-commerce search is hard to manage because there are a million different ways people can tell you what they want, and it’s hard to predict the words they’re going to use. Then, the language itself is complicated. For example, if you search for shorts, you might get a pair of short pants, but you might also see a short-sleeve shirt, a short skirt, a “skort” or any number of other things. This is happening because the word short and shorts have a common root, and the search engine can’t distinguish between short as an adjective and shorts as a noun. To make matters worse, what we normally call a pair of shorts is technically called just a “short.”
To deal with these issues, retailers set up rules, but manual rules might cause different problems. An example is a search for “leopard print.” In the results from a website I showed in one of the Lucidworks webinars, I get prints, as in wall art, with various animal images in my search results, but I don’t get any garments with a leopard pattern, which is what I wanted. This started when someone — probably someone focused on home décor — decided that the word leopard should be equivalent to the word animal but didn’t think about “leopard print” fabrics.
Tuning searches manually not only takes a long time to do in the first place, but once you’ve put in a manual curation rule, you have to maintain it as time goes on. Rules don’t last forever because products and searches change. You also have limitations on the number of rules you can put in. A manual process is painful and just not sustainable.
Q: AI and machine learning can process much higher volumes of data much faster than humans, but why doesn’t it make the same kinds of mistakes?
A: Machine learning is about pattern recognition and drawing conclusions based on patterns. Lucidworks uses what’s called signal ingestion or signal processing. Signals are all the user interactions — queries, clicks, add-to-cart, checkout, etc. — as well as all the profile and/or third-party data you have about a session. A signal is a broad concept, but there is a clear set of best practices.
The system isn’t “understanding” the meaning of the words; it is learning through search behaviors which words and combinations of words are important to determine the searcher’s intent.
Let’s say people are searching a site like Best Buy or Walmart for “dysentery.” The system doesn’t know that it makes no sense for a person to search for dysentery, which is an intestinal infection, on a normal big-box-store ecommerce site. But by going through enough sessions, the ML begins to see that people who search dysentery are buying Dyson vacuums. No matter how informed they are about the product or the customer, a human merchandiser would never think to create a rule like “dysentery=Dyson.” The pairing is not logical to human intelligence, but it works for real-life searches because the iPhone’s internal spelling correction changes the word Dyson to dysentery.
“a human merchandiser would never think to create a rule like “dysentery=Dyson.”
Q: Retailers have inventory they need to sell and promotional commitments to meet. They need to match products to the right buyer, not necessarily match the buyer to the product. How can AI help with that?
A: Those types of business needs are just another feature to engineer, and it can be done either at the ML model level or it can be done as a human override. When you build an algorithmic search experience, you simply want to reduce the number of decisions that humans are making.
With Fusion, the system sometimes suggests new rules based on the data, and the user can decide whether to accept it. Fusion also allows merchants to come up with rules so that they can achieve business goals, but they have to test them and experiment with actual searches. As a fellow speaker in one webinar put it, “Experimentation is the best way to make a data-driven decision.”
Fusion is powerful because it gives a retailer just-add-water-type solutions to tune common ecommerce searches, and those have been tested over millions of searches with numerous retailers. Fusion 4.2 provides a business rules manager that gives retailers the ability to build their own models and plug them into the Lucidworks framework to customize it.
Q: We’ve just touched the surface of how AI and ML can transform search for consumers and retailers. What are your final thoughts?
A: I spent the beginning of my career in web content management, where we were always trying to make companies more efficient. I like working in ecommerce search because the work tends to make companies more money. The reason is that search shows intentionality. People who search generally want to buy, and the old saying is absolutely true: If they can’t find it, they can’t buy it.
So, if your search is hiding relevant results, or if your relevant results are lost in a sea of irrelevant results, or if your search UX is messed up, then an investment in addressing these things will almost always pay off. Some of the companies that have implemented Fusion have been able to return their investment in a matter of days. It can be quite an enormous and quick return.
Marie Griffin is a writer and editor with extensive experience covering retail, technology, media and other B2B topics. She has held multiple editorial leadership positions, including editor/associate publisher of Drug Store News, and has been freelancing for web/print publications and marketers since 2001.
LEARN MORE
Contact us today to learn how Lucidworks can help your team create powerful search and discovery applications for your customers and employees.