How Semantic Search Helps Users Help Themselves
Learn how Semantic Search improves self-service and user experiences. When real-life interactions are limited, meet your customers where they are: online.
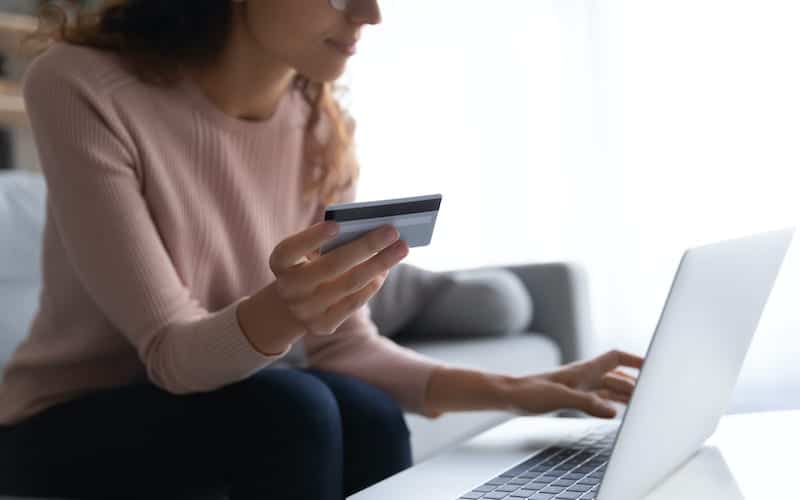
When it comes to information retrieval in the digitized world, meeting the need for user self-service is becoming the new measuring stick. In Justin Sears’ recent post, he explores how effectively we can replace in-person experiences with digital ones during the COVID-19 pandemic. One of the five steps he covers is the use of digital assistants to ease capacity issues. As customer retention and cost-control become increasingly important for businesses survival, so does self-service.
Users want to be able to interact with technology in the most natural and comfortable ways. The expectation is that you can type or vocalize queries in a natural, free-text way and that technology will understand not only your words, but your intent, and recommend relevant information.
This goes beyond what basic keyword search has been able to address in the past. Looking at search queries and understanding context (explicit or implicit) requires the use of sophisticated methods based on deep learning techniques. Semantic search as an umbrella methodology, has been successful in complementing keyword search and enhancing machine understanding of user intent and context.
How Semantic Search Works
Semantic search is the process of interpreting the intent and contextual meaning of queries and content. Unlike traditional keyword search, which only matches documents explicitly containing those keywords, Semantic search typically supports full natural language queries with a more human level of understanding.
One of the emerging and most powerful techniques for implementing Semantic search is based on using mathematical representation (embeddings) of text to understand its semantic meaning. Text embeddings can be applied to words, sentences and even phrases. Natural Language Processing (NLP) methods that use vectors to represent text have been researched and applied for quite some time. These methods allow for understanding how mathematically similar search queries can be from potential results and then ranking the results based on that similarity.
Applications of Semantic Search
Due to its versatility, semantic search is used to augment a variety of scenarios:
- Improvements to Traditional Text Search: Language interpretation, contextual/personalized interpretation, disambiguation, phrase and misspelling detection, etc. is possible with semantic search.
- Question Answering Systems: If a body of knowledge is available via frequently asked questions (FAQ), existing question and answer pairs, or just documents, semantic search models allows us to match the similarity of a question (asked in many different ways semantically) to the correct answers.
- Content-Based Recommendations: Commonly used in ecommerce, when users select certain results (like a product), semantic similarity is applied to deliver recommendations of products that have similar product definitions.
- Augmented Image Search: Retailers can take advantage of semantic search by using an ensemble of methods to hone in to the presentation of products: image content (object and color detection), product description (semantic search), existing tags and historical clicks (collaborative filtering). When all these methods converge, the relevance of search results is augmented substantially and can lead to a lift in conversions.
The Value of Self-Service in Information Retrieval
At the core of the definition of self-service is that users help themselves. If we look at the digital channel (mobile and web) the entire disruptive premise is that users are “helping themselves” more by using technology, and retrieving the right information to support the next decision is vital.
Companies that have prided themselves on offering superior experiences in human-to-human interactions, now have to reinvent how they can offer a similar experience at larger scale through a medium that allows users to self-serve in an easy and intuitive way. This assumes building automated intelligent systems that allow users to just ask a question, in the same way they would talk to a person over the phone, and get the right answer back.
Besides an improved user experience, there is a cost component that will be a factor for most companies. There are several studies, including this one from IBM, that attempt to approximate the cost burden from human-intensive customer service of inbound inquiries, as well as the advantage of automating and democratizing the retrieval of information through self-service question answering systems. To summarize IBM’s findings:
- Businesses spend $1.3 trillion on 265 billion customer service calls each year
- Phone interactions cost around $35-$50
- Text chat costs about $8-$10 per session
- It is realistic to aim to deflect between 40% – 80% of common customer service inquiries to automated frameworks.
- A drop in per-query cost from $15-$200 (human agents) to $1 (virtual agents)
The ultimate goal is to balance customer experience with costs. In the case of information retrieval, this can be done by using automation and intelligence in the search experience of your applications.
Deliver Smarter Answers with Lucidworks Fusion
At Lucidworks, we’ve been working with customers who want to improve the way they deploy chatbots and virtual assistants. By using a question answering system powered by semantic search and deep learning, customers can improve user experiences and reduce the human-intensive cost of delivering those experiences.
To learn more about Lucidworks Fusion for customer and employee support, contact us today.
LEARN MORE
Contact us today to learn how Lucidworks can help your team create powerful search and discovery applications for your customers and employees.