Machine Learning Powers Digital Transformation
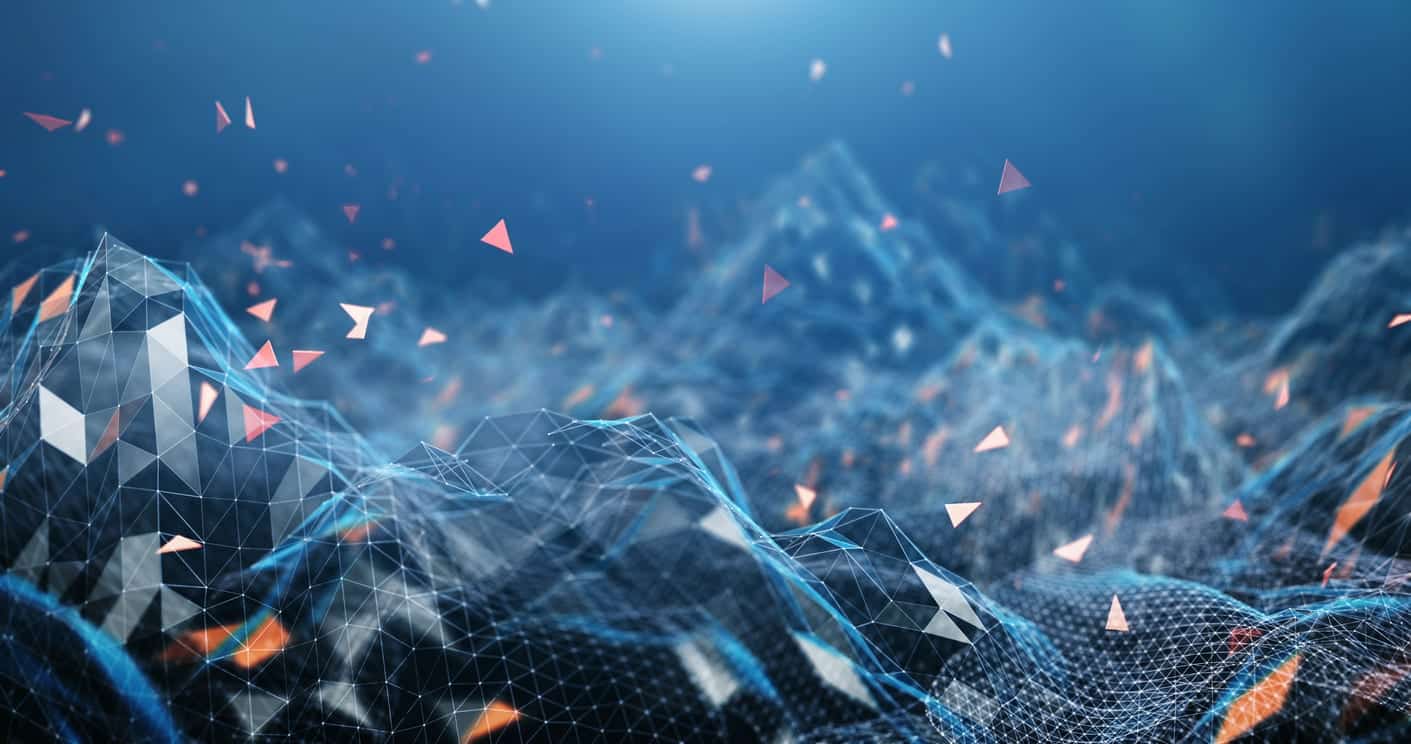
Understanding your customers is key for any organization. This was true at the beginning of time and is twice as true now. Good service isn’t just a shopkeeper with a great smile, remarkable memory and a knack for observation. It is a computer system collecting data and applying algorithms.
Collecting the data and knowing what customers want is great, but if an organization can’t adapt to their customers’ needs then all of the data in the world won’t help make the deal. The speed required for this is measured in clicks and seconds, not months. Digital transformation means automating all back-office processes to work in Internet time.
Machine Learning Is Key to Digital Transformation
In its infancy, online retail meant tons of manual updates by business people and frequent code rewrites by developers. These days, there simply isn’t a way to create or change enough code, rules and manual processes to adapt quickly enough to both customers and increased supplier and distribution chain complexity.
Is product in stock? If not, when will it be available? Where is it coming from? Pulling data from multiple systems can be extremely difficult. With so many customers and products, this has to be done in new ways.
Data scientists are using new machine learning techniques, combined with the expertise of product merchandisers, to adapt to the changing behavior of customers and their increasingly complicated supply chain.
Implicit Data Through Signals
Customers give off signals when they click, query, add something to a cart. They give off signals when they buy something. They give off signals when they don’t buy something, don’t click on something, or abandon their carts.
It is easy to understand that customers who search but don’t click on results, probably aren’t finding what they are looking for. Figuring out why they don’t click and what they really want, is a pattern problem.
Understanding other customers who clicked or didn’t click on similar things leads to a better understanding of what other searchers may have wanted. The process of understanding that behavior is usually automated so sites can identify similar customers and show them what they want.
Unstructured Data
However, looking at implicit data isn’t enough. Fortunately, there is a lot of explicit data stored in unstructured data written in natural language. We gather this data from what customers type in search boxes and what they write in to reviews, comments, and support requests. And, this data can live in different places like reviews on retailers’ or third-party sites, such as Good Reads or Stack Overflow.
Natural language processing techniques are one way of adding structure to this data, but, frankly, these never worked all that well. They required a linguist, a developer, and a data scientist — which sounds like the beginning of a bad joke.
New techniques give up on perfectly structured knowledge graphs and instead rely on neural networks, deep learning, and machine learning techniques to improve understanding over time. Techniques include sentiment analysis and neural ranking models for information retrieval which “use shallow or deep neural networks to rank search results in response to a query,” according to “Neural Models for Information Retrieval.”
This is also true on the supplier and distributor side. Everyone has a different way to describe things. Figuring out exactly what a product is and where it should be categorized is a delicate science — except some deep learning techniques make it possible to classify products and determine that “oceanic hue” also means “blue-green.”
Results won’t be perfect — you’ll still need to combine it with rules — but it will be possible to do it at the speed of modern retail and with greater overall accuracy. Retailers such as Bluestem Brands have been dealing with this problem in new and innovative ways.
Data and Machine Learning Is People
“First they ignore you. Then they laugh at you. Then they fight you and then you win,” a quote misattributed to Gandhi sums up not just his political strategy but also to some degree the Gartner Hype Cycle for emerging technologies. The trouble with both is that the fear and hype around AI technologies such as machine learning and deep learning is based on a fundamental misunderstanding.
Machine learning will not replace merchandisers and human expertise. On its own, it does nothing. Absolutely nothing. Subject matter experts supply the business understanding, data scientists select or create the right algorithm, and data engineers structure the data and apply the algorithm. But software automation can help.
For instance, you don’t need a data scientist to cluster customers by their signals because your software provider already hired one and packaged it up for you. However, every retailer should have a team that looks for new opportunities to apply these algorithms to new problems throughout the business. Building that data science competency center is critical to success.
Building the Machine (tl;dr)
Digital retailers are having a lot of success; however, only the ones who remember the secret that allowed the oldest shops to succeed: personalized service and adapting to change. Everything needed to provide that at Internet scale exists in the data that customers and suppliers provide.
Using new machine learning and deep learning techniques is the key to making personalization and adaptation work at the speed of the modern digital world.
The most successful digital retailers will combine these techniques with the expertise of merchandisers and data scientists in order to perform digital transformation and personalization throughout their operations.
LEARN MORE
Contact us today to learn how Lucidworks can help your team create powerful search and discovery applications for your customers and employees.