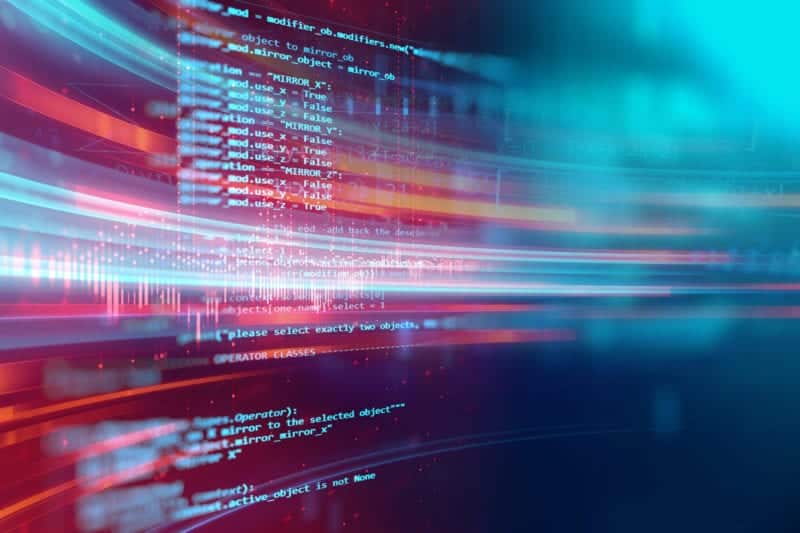
Separation of Risk From Insight
This separation of managing risk from deriving insight made a lot of sense historically. The teams charged with managing various portions of data risk were generally part of a legal, security, or compliance function. They employed technologies that tended to be purpose-built for narrow functions, such as books and records systems, data loss prevention, asset management applications, litigation collection tools, etc.
This approach addressed discrete obligations, often at a system level, or executed through ad hoc processes. In the meantime, lines of business and marketing teams employed ever more sophisticated data analytics and processing to derive insight and collaborate across large volumes of information.
In their quest for insight and collaboration, employees have bought data, uploaded it to cloud BI tools, inadvertently moved data where it does not belong, or moved it outside of appropriately controlled environments.
The result is that over the last decade, companies in even lightly regulated industries have found themselves as either victims of data breaches or subjects of government investigations into use or access of “private” information.
For example, in 2018 security researchers identified unsecured Amazon S3 access to passport and associated data for 118,000 FedEx customers from an environment setup by a company Amazon had acquired years earlier. Cambridge Analytica did not execute an orchestrated cyberattack at Facebook, but rather exceeded its authority for access and use of personal data.
Two notable breaches in 2019: Toyota’s Japanese offices jeopardizing data of 3.1 million car owners and the U.S. Federal Emergency Management Agency’s exposure of data on 2.5 million disaster survivors. – Cybersecurity Ventures.
This surge in breaches and exposure risk was driven in part by the explosion in data volumes, along with the ease with which information could be disseminated across many channels and stored outside of corporate controlled networks. This also highlighted a key change from historic models to manage information risk; it was no longer limited to system-level management and control, but rather is based on the nature of data itself.
One great irony is that several of the most well-publicized issues associated with privacy-related information arose because of the tremendous capabilities developed in data aggregation and analytics. Social media platforms, websites, enterprise systems, and search applications collect and allow correlation of huge volumes of data to provide unprecedented insight, but also creates risk hardly contemplated 10 years ago.
The dynamics associated with pervasive data access and analysis have also increasingly moved risk closer to where business and services are delivered or where collaboration occurs, and outside of traditional records, ERP, or internal systems. The latter trend means locking down formal processes or relying heavily on static systems and workflows to identify and mitigate information risk, will likely be incomplete.
Methods for Evaluating Risk
The idea of evaluating risk associated with data itself is inherent within any number of regulations and industry best practices. As representative examples:
- The E.U.’s General Data Protection Regulation (GDPR) represents a codification that the risks are associated with the information itself, (versus systems, networks, or technologies).
- ISO 27001 establishes standards to inventory and classify information assets (8.2.x)
- The National Institute of Standards (NIST) has a number of frameworks that incorporate a requirement to categorize the nature data processed, stored, or transmitted.
- State-level privacy laws, including California’s Consumer Privacy Act (CCPA), define a broad set of sensitive information and risk based controls
- The HIPAA Privacy Rule establishes standards that define personal health information and obligations to manage associated risk.
Assessments Are More Than Policy
Many organizations have undertaken some form of data mapping exercise as a means to begin planning and establish system level controls. These exercises often take the form of surveys, interviews, or general descriptions of data that is commonly stored, processed, or shared. We see clients that have asset or system level inventories that generally describe the type of information that should be there; but often do not have a means to validate if that remains true over time.
We also see organizations taking their security and data governance requirements seriously, but much of their work to date has been at the policy level, or are based on access controls and logs, which is certainly an important element. However, many do not broadly employ solutions to verify what data is present, and the nature of the underlying information contained within a given data source, and whether it conforms to their policies. As a result, system or access level controls and audit logs will prove ineffective, if sensitive or restricted data finds its way to a source where it does not belong.
Managing data risk involves layers of technology and processes; to date this has often focused system and network level controls. A data risk assessment allows an organization to (1) directly ascertain whether proscribed data is contained within a document, a data source, geography, or a communication channel; and (2) provide ongoing monitoring as a means to audit and measure adherence to defined controls.This is a critical missing layer for many companies.
An effective data risk assessment requires the ability to access the data, index and extract pieces of information across multiple dimensions, and finally appropriately analyze the data for patterns and types of data that create potential risk. This may include sensitive identity/personally identifiable information (PII), special categories under GDPR, health information, PCI, or other forms of confidential internal data.
I have worked with solutions that tried to deal with this either by deploying massive, propriety infrastructures, or building extensive integration into sources that were obsolete before ever completed. They also lacked ability in processing and analyzing diverse types of information, leading to imprecise and noisy results.
Functional Specification for Risk Assessment Platforms
- A platform that enables you to execute data risk assessments must have
- An open and scalable platform that allows rapid evolution, ease in integration, and limits proprietary risk
- A breadth of machine learning algorithms and AI within the platform, that are relevant for Natural Language Processing, entity extraction, document clustering, and categorization
- A comprehensive set of supported (and regularly updated) set of connectors to the most common sources of data, providing access and integration where data is stored, processed, or shared
- The ability to define via regular expressions (RegEx) the most common patterns associated with PII, PCI, Account and ID related data, and similar
- Flexible index and query pipelines allow for using a combination of machine learning, pattern (RegEx), and text base analysis of data sources, which provides a deeper and more precise set of results
Learn more and watch the “Enable Insight Driven Data Risk Assessments With AI” webinar featuring Simon Taylor and me here.
In my next blog, I will layout in more detail how clients can start to use Lucidworks, the same platform many are using for information insight, to start assessing information risk.
Managing Partner of SOHO2, a consulting firm providing advisory services, and delivering innovative solutions for information risk and insight. George has extensive experience with legal and compliance solutions, and information analytics. He has a J.D. from the DePaul College of Law, and is admitted to the Illinois State Bar, and the Federal District for the Northern District of Illinois.
LEARN MORE
Contact us today to learn how Lucidworks can help your team create powerful search and discovery applications for your customers and employees.