Digital Transformation Lowers Risk of Oil and Gas Exploration
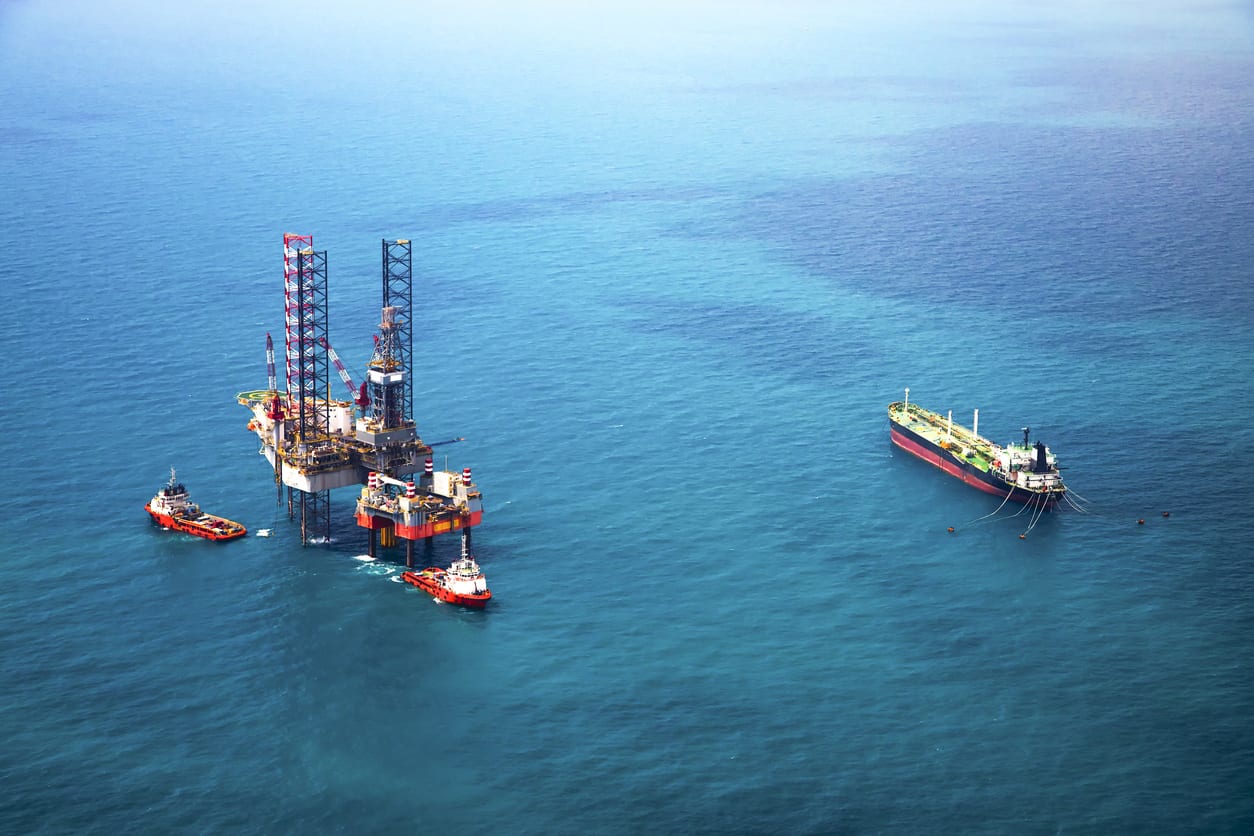
For major oil and gas producers, the risk associated with exploring potentially lucrative oil prospects is often accepted as the cost of doing business. For example, in 2015, after eight years of planning and drilling, Shell announced a $4 billion write down as a result of a single failed exploration project in the Arctic.
In recent years, the application of technologies such as machine learning (ML) and artificial intelligence (AI) have helped forward-thinking operators lower their risk by providing exploration teams with valuable insights about where to drill. In such cases, advanced analytics are used to search and interpret decades worth of untapped information — much of which is locked in data silos — to make more informed exploration decisions and avoid multi-billion dollar mistakes.
Despite these benefits, the majority of exploration and production (E&P) companies continue to rely on traditional methods of data retrieval and analysis. However, as operators search for ways to increase efficiency and profit margins in the “lower for longer” environment (i.e., with crude oil prices fluctuating between $50 – $75 per barrel), the value that digital transformation can provide is becoming harder and harder to ignore.
The Role of Search in Upstream Oil Exploration
The primary objective of upstream geophysicists and supporting data scientists is to identify and model hydrocarbon resources that can be exploited cost-effectively. Today, the vast majority of exploration takes place offshore in deep water. In this environment, drilling of a single exploratory well can cost upward of $150 million. With documented success rates of around 20 percent, it is critical that personnel responsible for analyzing geology and identifying locations to drill are able to make an informed decision based on all relevant data.
And therein lies the problem.
In most cases, the information required to make these decisions resides in a variety of locations and file formats (e.g., text files, well logs, GIS files, images, etc.). In the case of large E&P companies whose operations span decades and millions of surface acres, internal databases often consist of highly disparate, siloed, and unorganized data. This makes it difficult for engineers and geophysicists to find and access the right information using conventional search applications. Common issues companies encounter include:
- Available, relevant data takes too long for users to find and access (i.e., poor search functionality)
- Inconsistencies in how data is labeled and tagged
- Some data is so disparate that decision-makers are not aware that it even exists
- The location of information and data related to past projects resides in the minds and/or private notes of experienced personnel and is inaccessible to new employees
- LAN (or GIS) files constitute a significant portion of wanted yet unsearchable data
- Efforts to find and access data are often duplicated by separate teams, wasting time and resources
Leveraging Search Analytics
The upstream oil and gas industry is addressing these challenges by making data applications more intelligent. In one instance, a major E&P operator had more than 10 million surface acres in its portfolio and was focused on driving digital transformation at a massive scale. An important part of that initiative involved applying advanced search analytics and machine learning to change the way exploration teams could find and access upstream data.
The objective of the project extended beyond simply providing users with a faster way of locating relevant information. It was about creating a compelling data experience by using analytics to connect information silos, create an agile catalog of context and relevance, and enable users to extract relevant business knowledge across all historical databases.
To achieve this, the operator deployed an AI-powered search and discovery application based on Lucidworks Fusion, which provided the following advanced capabilities:
- Collecting documents to be processed while maintaining information security and OCR as necessary
- Analyzing documents via natural language processing (NLP) in English, French, German, Russian
- Analyzing each file content to determine most likely location(s) based on country>basin>block>field>well hierarchy
- Creating relevancy by classifying documents based on their content
- Enabling information retrieval across data sources
- Refining relevancy with user rating of retrieved documents
- Visualizing results via Map, word cloud type interface for dynamic filtering, and timescale filtering
- Facilitating discovery of new information via triggers based on specific interests saved by users, as well as Google-like “you might be interested in …” suggestions based on browsing history and popular queries
A key component of the intelligent search application is its use of machine learning, which allows it to continuously improve, based on the individual input from the user.
With the deployment, the E&P operator can answer user queries and solve complex search and relevance problems, neither of which was possible using its existing search platform. This enables exploration teams to leverage more effectively the wealth of upstream data that has (and is) being generated by giving them the ability to quickly and accurately retrieve information when they need it, which ultimately leads to better decision-making.
The Road Ahead
Digital transformation continues to serve as a powerful driver of success for industrial enterprises. While the oil and gas industry has not been immune to this trend, it historically has lagged behind others on the digitalization maturity curve. However, this is changing as organizations, particularly in the upstream sector, face increased pressure to reduce costs and minimize risk.
In the near-term future, the primary focus for forward-thinking operators will be on leveraging big data and analytics to improve decision making and streamline existing business processes. During that time, the role of ML and AI in upstream operations will undoubtedly grow immensely.
Alex Misiti is a former civil and environmental engineer turned freelance writer who focuses on topics relating to oil and gas, renewable energy, IIoT, and digital transformation. He is the owner of Medium Communications, LLC.
LEARN MORE
Contact us today to learn how Lucidworks can help your team create powerful search and discovery applications for your customers and employees.