Explainable AI & the Black Box in Deep Learning
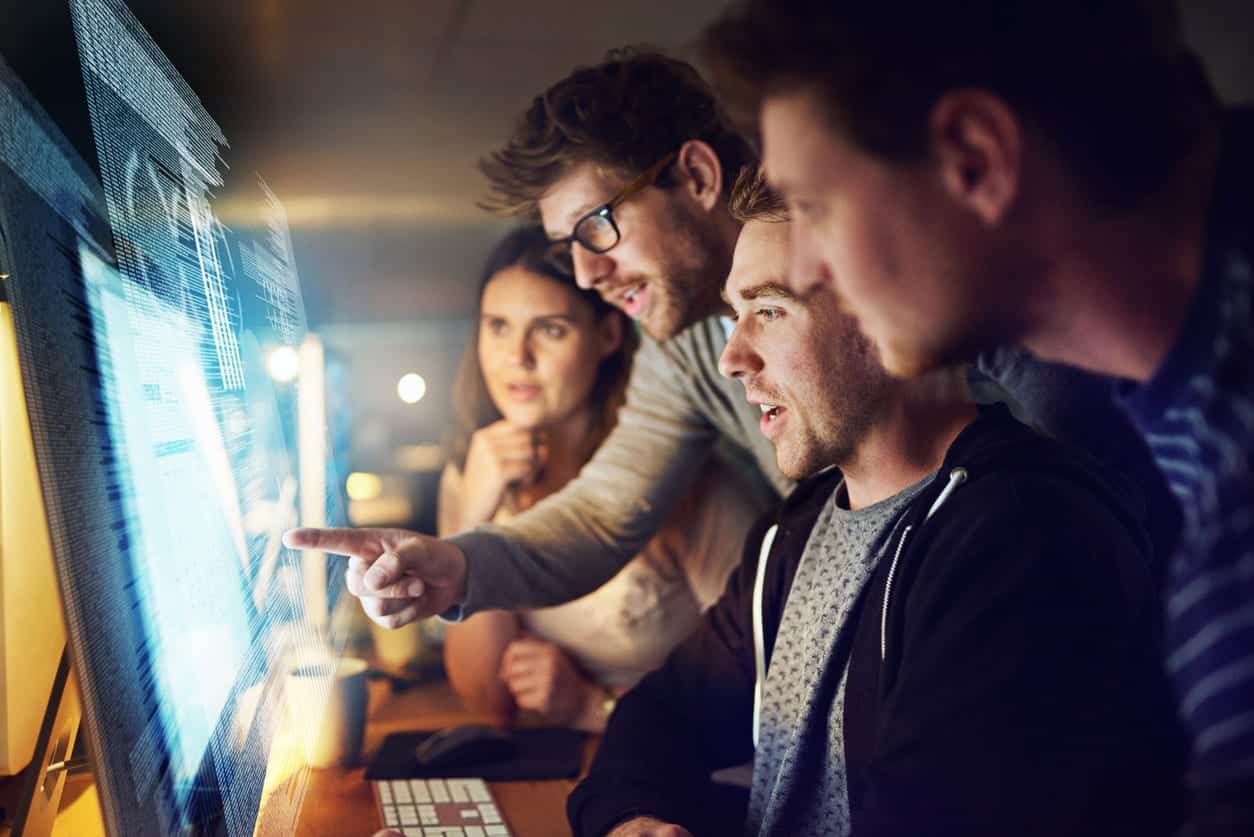
Walking through conferences it seems everything is now fortified with AI. But what does that mean? And is that vendor’s offering really for you? It depends.
Advantages of Machine Learning
There is no debate that machine learning rapidly produces highly reliable and sophisticated insights — when input is valid and output is thoroughly tested. Users train a mathematical model with representative examples of training data and compare the results with expected targets for that data. The machine infers classification from the human classified data set.
Multiple rounds of testing with different data sets (some of which are subsets of earlier data) serve to tune and then finalize a model. Because users compare and tune the results of the machine learning classification with the results of the human classification — a process called supervised learning — the results are consistent and trustworthy.
The more data that goes through the system — the smarter the application becomes. Or so one would think.
What happens though, when the data is not valid, when the content changes over time, or when users expect changes that are not contemplated in the original training data?
The Risk to Enterprises
In 2016, the public-interest research organization ProPublica discovered that a for-profit enterprise’s AI algorithm provided racially-biased output while scoring Broward County, Fla., arrestees on their likelihood to commit future crimes.
Also in 2016, Microsoft took its AI chatbot Tay offline after multiple episodes in which it published racist, misogynist, and drug-related tweets that were based upon information that Tay learned from other Twitter users.
And as recently as 2018, individuals have been killed by autonomous motor vehicles. In two separate cases involving Tesla and Uber, vehicle software misjudged the circumstances leading up to a fatality, and then human backup drivers – who perhaps trusted the software too much – failed to intervene in time.
Unaccountable AI
In cases of AI error large and small, questions of incident prevention, investigation, accountability, and liability are obscured by AI’s black box, in which automated decisions are made at light speed through elaborate and sometimes unforeseeable algorithmic interactions.
In regulated industries and public services such as health care, such snafus present professional, legal, and financial risks — even risks to human life. Black boxes make it difficult for managers to assign responsibility for issues, resolve liabilities, and support optimal decision-making.
But if AI black boxes are really that troublesome, why use them? What can your organization do to resolve these risks and exploit trustworthy AI-derived business insights?
As is typical with almost everything associated with AI, there is confusing, almost paradoxical, use of the term black box that causes confusion.
In the last two instances above, the black box refers to the layering of algorithms necessary for what is called deep learning. The organizations’ own data scientists derived the algorithms – but essentially lost control of how they interacted within a neural network, causing the unintended consequences inherent in a black-box approach.
Challenges With the Black Box
While deep learning black boxes are important to explore, it is the instance that ProPublica uncovered above that is the more typical type of black box that companies are faced with today. In most cases they are using a trusted vendor that is now fortified with AI. These are desirable for organizations or departments that don’t have data scientists — and are excited about automating repetitive tasks.
But a black box may lead organizations to adopt AI models that may lead to undesirable outcomes. Unfortunately, you don’t have control over those results, and at best, you may be able to override some results, but only if you know the aberrant results and the desired one.
This may be less problematic if the system is trained exclusively with your data. But, again, with organizations and departments wanting turnkey solutions at the lowest possible price, vendors are creating cloud solutions that commingle your data and other companies’ data to determine outcomes.
Further, many of the black-box solutions are cloud based and use the same algorithm across all of their clients data sets. So the application is being trained — but perhaps not in the way domain experts might want.
In retail, for example, black box merchandisers will boost certain types of products — based on the behaviors across all retailer customers. This might be great if you are selling more commodity products, but certainly not acceptable if you cater to more niche customers or if you are trying to be more than a vendor and create a “destination” site and generate a “stickier” experience for your customers..
AI Requires Trust — and Trust Comes From Control
In industries such as health care, doctors, patients, and insurers must have confidence that an AI prediction is rooted in both sound and unbiased source data. These data sources also need to reduce unknown variables as well as take into account any known or plausible symptoms and risk factors. If an AI process has secretly assumed heretofore unrecognized markers for a disease, this assumption must be explained to doctors and scientists, and they must confirm the validity of that assumption.
In the case outlined by ProPublica, jurisdictions across the nation are using a variety of tools to determine the recidivism rate of individuals convicted of crimes. Again, economics can necessitate that a vendor provides machine learning services instead of training the system on your own because of resource scarcity or the lack of jurisdictional data sets appropriate for training. Two vital questions that must be asked are: how is that data being trained, and what assumptions are being made?
Napa County Superior Court Judge Mark Boessenecker said he finds the recommendations helpful – but not infallible. “A guy who has molested a small child every day for a year could still come out as low risk because he probably has a job,” Boessnecker told ProPublica. “Meanwhile a drunk guy will look high risk — because he is homeless.”
Despite efforts to say we are relying on the math — there is a human bias that can come into play. Some data is weighted more heavily than others. Having a job may indicate an individual is less likely to commit a crime than not having one but is that the proper metric to weight so heavily?
(Criminal populations may behave differently in different parts of the country. An area with high homelessness or high unemployment, may not be the predictors of say, a locale with the inverse.)
Explainable AI
A term that is evolving to be the opposite of black box is explainable AI (XAI). And while XAI has nuanced meanings in deep learning, we can use the term to describe how you can have confidence in the results.
Some of the characteristics of explainable AI include
- Having control over or access to the algorithms that go into training
- Knowing and controlling the data that is being used
- Using a domain expert to help train the model with a known data set
- Understanding any biases or assumptions that go into the model
Impact of Explainable AI
It is highly unlikely that AI will ever achieve 100 percent certainty. But in industries where decisions are life or death — or life-impacting –, explainable AI will provide far greater certitude over your results.
There is another factor, too. When you use a cloud provider, your data is helping that vendor’s product become smarter, and you are becoming reliant on training data from another company that may not be as trustworthy as you’d like. You literally are using your intellectual property to build equity in someone else’s product and are becoming reliant on others’ data at the same time.
What we are seeing is that algorithms are the new IP — and you might not want to hand yours over so easily.
LEARN MORE
Contact us today to learn how Lucidworks can help your team create powerful search and discovery applications for your customers and employees.