AI Shines Path For Customer Journey Mapping
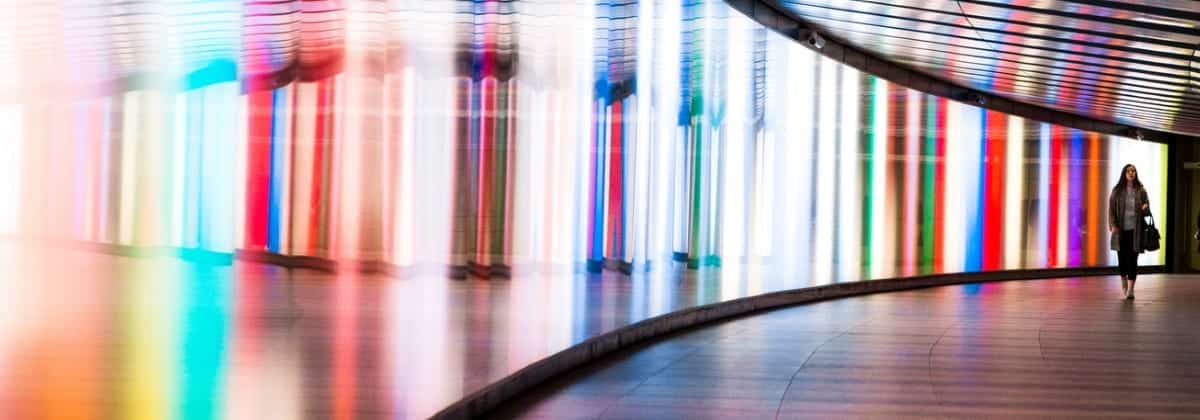
In a world where it is so very easy to spend money in any form, consumers can be forgiven for thinking that accessing any and all financial services would be painless. And in a time when financial institutions have a wealth of information on customers, banks should be able to make it so. That’s where artificial intelligence (AI), and machine learning in particular, is making great gains.
One of the great frustrations of the financial services business has been the inability to recognize important pain points in customer relationships. Frustrating interactions — unclear instructions on loan applications, or unnecessarily onerous requirements to cash checks — can cause customers to give up on looking for a new product or to consider looking for a new financial services provider altogether.
Even when banks do identify barriers for customers, understanding their impacts is difficult, if not near impossible.
Not so long ago, it was virtually impossible to track individual customer behaviors. A frustrating paper loan application landed in a trash can, offering no evidence to the bank of why it was abandoned. (In fact, the bank may never even have known that a customer was considering a loan in the first place.) Banks had no way of tracking how many times a customer tried to cash a check but left a branch in annoyance because a second form of ID was sitting at home. These behaviors were offline, effectively hidden.
Hoarded Data Was Dark Data
But let’s be real — banks have had lots of other types of customer data because it has been part of the very nature of the business to collect and retain vast amounts of information. According to the Washington Post, the oldest piece of writing discovered in London is from 65-80 AD, and it was — wait for it — an IOU.
Since the time when accounts were kept in huge handwritten ledger books, through the day of the passbook savings account and now, a few decades deep into the digitization of virtually all customer data, financial firms have necessarily been the repositories of enormous amounts of data about their clients.
While the industry indubitably has scads of data, it has had little ability to use it.
Oh sure, very customer-specific queries can be handled with a fair amount of ease. For example, if Ms. Jones was asking for a line of credit, the bank could look at its records to see how reliable she had been about paying her mortgage.
But they couldn’t easily look across customer accounts to see how people similar to Ms. Jones had fared with a line of credit. Or, crucially, to identify customers like her who might respond positively to a proactive offer of a credit line.
More Data, Few Insights
That’s changing. “Credit card companies have been collecting all this stuff about us since there were credit cards. The information has been available. It has just been really, really difficult for most organizations to pore through it and actually make sense of it,” said Greg Kihlstrom, senior vice president of experience at Yes&, a digital marketing agency in Alexandria, Va. “I think that’s the whole big data thing, what that gave us was just … mountains of data. Even then, most organizations didn’t really have a plan for it,” said Kihlstrom.
Then the digitalization of workflow began to happen — and an influx of even more data was available.
Indeed, the amount of data financial firms can accumulate is an order of magnitude larger than it was just 10 years ago.
When a customer begins a loan application these days, it’s probably online. It’s a relatively simple matter to figure out precisely where in the process a potential borrower abandoned it. When a bank loses customers today, it’s far easier to search transaction histories and try to understand why they might have been dissatisfied.
Until recently though, as Kihlstrom notes, banks’ abilities to collect ever larger amounts of data on customers had largely outpaced their ability to analyze it. But things have changed.
“Now we’re actually at a point where companies’ data mining is mature enough — because of AI — where we can actually start using big data,” he said.
Machine Learning Allows Easier Data Mining
Advances in artificial intelligence and machine learning have made it possible for banks to take the data they already possess, and mine it for insights that can help them address customer pain points in a systematic way.
Customer experiences — both good and bad — are also commonly referred to as the customer journey. This highlights the fact that most clients’ interactions with a financial institution take place over time, involve interactions with different departments within the company, and may produce data points that exist in separate silos with little or no intercommunication. In other words, banks act like almost every other enterprise — they store data in all sorts of places and one hand may not know what the other hand is doing.
According to Forrester Research, the ability to map the customer journey is essential to a financial institution looking to improve its customer experience, and it necessarily involves a heavy reliance on data analysis.
Journey Mapping Key to Next Best Action
In a recent report, the firm found that financial services firms ought to use analytics to drive a more data-driven approach to mapping customer journeys.
“Customer-led companies don’t just collect information on customers — they also use it to proactively engage them,” the report said. “Use data such as web analytics, customer feedback surveys, complaint and customer satisfaction data, or contact-center call log verbatims to analyze customer interaction data across silos, fix cross-touchpoint issues, uncover operational bottlenecks, build optimal cross-touchpoint customer experiences, and drive desired behaviors.”
In the same report, Christopher Cox, chief digital officer at USAA, said that “too often, the creation of new digital capabilities are treated as projects, with a beginning, a middle, and an end.” And Grant Ingersoll, Chief Technology Officer for Lucidworks said one of the challenges is that insight initiatives have often been projects instead of a new way of doing business — and this leaves gaps. But now “we see our banking customers leveraging AI-driven search and analytics to power customer 360 views that encompass all the touch points a customer has with the bank.”
AI-driven search is a nebulous marketing term that virtually every vendor uses. Ingersoll prefers the more specific “machine learning.”
“Banks are using machine learning systems to integrate a large number of data sources — from transactional information to a client’s preferences for coffee — and make the data accessible and actionable,” Ingersoll said. “Machine learning algorithms and other statistical techniques are constantly scouring the data, classifying it, relating it, and examining it for trends and anomalies at the individual level.”
This process also enables smarter recommendations for users and better reporting for employees on core business objectives.
Perhaps most importantly, the “most advanced banking customers leverage the system to enable ‘next best action’ analysis, which proactively informs users with information learned by the system,” Ingersoll said.
AI Drives Automation
Advanced analytics also make it possible to automate processes that were once thought beyond the reach of AI. “Robotic Process Automation (RPA) is beginning to automate the multitude of repeatable processes across banking, for example, a customer requests a change of address,” writes Tony Farnfield, the London-based country lead for BearingPoint.
“Because of the many legacy systems traditionally found within a firm, this change request often results in the required update of six or more records from the CRM system, with a lengthy process ensuing for the customer,” he explained. “The automation of this process would contribute to significant savings of time and effort for both the firm and the customer, ultimately leading to an improved customer experience.”
This extends to the other elements of the customer journey as well. It is becoming clear that AI-enhanced chatbots are going to play an increasingly major role in the customer service operation of large financial services firms in the near future.
Proceed With AI — But Remember the UX
Integrating AI into the customer-facing elements of the business won’t come without risks, though, warns Jim Marous, publisher of the Digital Banking Report. What may look like a convenient efficiency to a bank, could look … creepy to a customer.
“It is important for digital solutions to be focused on building great experiences as opposed to simply being used to reduce costs or increase ROI,” he writes. “While consumers are increasingly aware and satisfied by AI-enabled experiences, they also expect a humanized engagement and a human presence to better enable interactions across the entire customer journey.”
In any case, there can be little doubt that as financial services firms increasingly move to streamline the customer journey, artificial intelligence and analytics will play a key — perhaps the key — role. And the question of how to handle it may confront industry leaders sooner than they think.
“Things are going to get a lot more automated a lot more quickly,” said Nihlstrom, of Yes&. “Now that we actually have the ability to make use of all this big data that people have been talking about for at least a decade, I think we’re going to make really, really quick progress on automating a lot of the jobs,” he added.
“The world is going to look very different in five years even for such a risk-averse industry.”
Learn More
- Download Transform the Financial Advisor Experience With AI
- See Lucidworks Fusion’s customer analytics capabilities
- Contact us for more information
Rob Garver is a DC-based financial services reporter.
LEARN MORE
Contact us today to learn how Lucidworks can help your team create powerful search and discovery applications for your customers and employees.